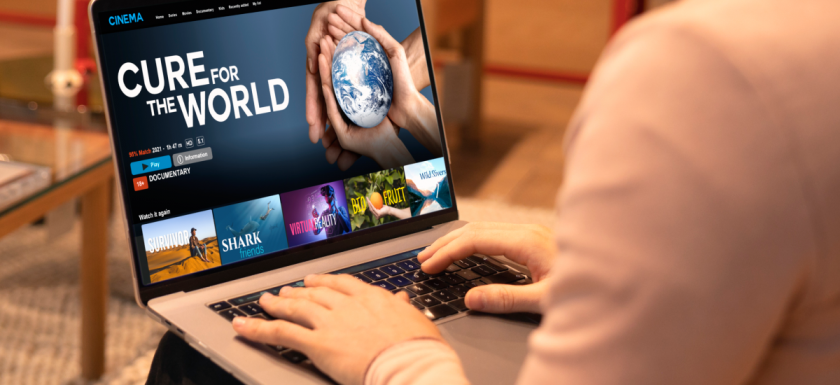
Source: Freepik
Copyright: freepik.com
Source image URL: https://www.freepik.com/free-photo/digital-nomad-working-remotly-their-project_21795560.htm
License: Licensed by JMIR
CONTACT: Lois E. Yoksoulian, Physical Sciences Editor 217-244-2788, leyok@illinois.edu.
CHAMPAIGN, Ill. — New research led by data science experts at the University of Illinois Urbana-Champaign and United Nations Global Pulse found that there is no strong evidence that YouTube promoted antivaccine sentiments during the COVID-19 pandemic.
The study, published in the Journal of Medical Internet Research, performs an algorithmic audit to examine if YouTube’s recommendation system acts as a “rabbit hole,” leading users searching for vaccine-related videos to antivaccine content.
For the study, the researchers asked study participants to intentionally find an antivaccine video with as few clicks as possible, starting from an initial informational COVID-19–related video posted by the World Health Organization. They compared the recommendations seen by these users to related videos that are obtained from the YouTube application programming interface (API) and to YouTube’s Up Next recommended videos that were seen by clean browsers without any user-identifying cookies.
The team then used machine learning methods to classify antivaccine content, analyzing more than 27,000 video recommendations made by YouTube.
We found no evidence that YouTube promotes anti-vaccine content to its users. The average share of anti-vaccine or vaccine hesitancy videos remained well below 6% at all steps in users’ recommendation trajectories. [Margaret Yee Man Ng, assistant professor, Department of Journalism and Institute of Communications Research, University of Illinois Urbana-Champaign; lead author]
The initial goal of the research was to better understand YouTube’s famously opaque techniques for content recommendations—going beyond querying the platform’s APIs to collect real-world data—and whether these techniques funnel users toward antivaccine sentiments and vaccine hesitancy.
We wanted to learn about how different entities were using the platform to disseminate their content so that we could develop recommendations for how YouTube could do a better job of not pushing misinformation. Contrary to public belief, YouTube wasn’t promoting anti-vaccine content, however the study reveals that YouTube’s algorithms instead recommended other health-related content that was not explicitly related to vaccination. [UN Global Pulse Researcher Katherine Hoffmann Pham, coauthor]
The videos that users were directed to were longer and contained more popular content, and attempted to push a blockbuster strategy to engage users by promoting other content that was reliably successful across the platform [Margaret Yee Man Ng]
The study also allowed the researchers to examine how users’ real-world experiences differ from the personalized recommendations obtained by querying the YouTube API’s “RelatedToVideoId” field, which is designed to help programmers search for related content on the platform, or using clean browsers, which replicate the experience of a new user visiting YouTube with no search or view history and are often used to study the platform’s recommendation system.
The study reports that the watch histories of users significantly affect video recommendations, suggesting that data from the API or a clean browser do not offer an accurate picture of the suggestions that real users are seeing. Real users saw slightly more provaccine content as they advanced through their recommendation trajectories. In contrast, searches performed by the API or clean browsers during the study were drawn toward irrelevant recommendations as they advanced.
I think one benefit of this study relative to others is that it proposes a relatively lightweight methodology to gather real data on how people navigate through Youtube’s video recommendations. So unlike the APIs, which will just sort of randomly suggest new links, the users can critically review the links and pick one, which mimics the behavior that many people would use on YouTube in reality. [Katherine Hoffmann Pham]
Understanding recommendation systems is important because it promotes transparency and holds them accountable. This helps people understand the choices being made for them by platform designers. [Miguel Luengo-Oroz, professor, Telecommunications School, Universidad Politécnica de Madrid; coauthor]
Margaret Yee Man Ng is also affiliated with the Computer Science department, the National Center for Supercomputing Applications, and the Center for Social and Behavioral Science at the University of Illinois.
Editor’s notes:
To contact Margaret Yee Man Ng, call 217-300-8186; email ymn@illinois.edu.
Original article:
Ng YMM, Hoffmann Pham K, Luengo-Oroz M. Exploring YouTube’s Recommendation System in the Context of COVID-19 Vaccines: Computational and Comparative Analysis of Video Trajectories. J Med Internet Res 2023;25:e49061
URL: https://www.jmir.org/2023/1/e49061/
DOI: 10.2196/49061
About JMIR Publications
JMIR Publications is a leading, born-digital, open-access publisher of 30+ academic journals and other innovative scientific communication products that focus on the intersection of health and technology. Its flagship journal, the Journal of Medical Internet Research, is the leading digital health journal globally in content breadth and visibility, and it is the largest journal in the medical informatics field.
To learn more about JMIR Publications, please visit https://www.jmirpublications.com or connect with us via YouTube, Facebook, Twitter, LinkedIn, or Instagram.
If you are interested in learning more about promotional opportunities, please contact us at communications@jmir.org
The content of this communication is licensed under the terms of the Creative Commons Attribution License (https://creativecommons.org/licenses/by/4.0/), which permits unrestricted use, distribution, and reproduction in any medium, provided the original work, published by JMIR Publications, is properly cited. JMIR is a registered trademark of JMIR Publications.